A race is underway amongst the largest technology companies (collectively referred to as the Magnificent 7) to create the first ‘Digital God’ - an all-knowing, all-seeing large language model (LLM) that could unlock tens of trillions, even hundreds of trillions in value. Sounds like hyperbole doesn’t it, but according to one investor – Gavin Baker, managing partner and CIO of Atreides Management – it’s a reality the people who are in control believe will happen and they’re locked in a race to be the first to create it and failure to do so would pose an existential threat to their company.
Before the launch of ChatGPT, the 'Mag-7' were competing in distinct lines of business with their own technological innovations that had little crossover between them. That changed when OpenAI’s ChatGPT LLM exploded onto the scene bringing AI to the forefront of everyone’s imagination. Recognising its potential, 'Mag-7' companies leaned into this technology in a bid to create their own digital ‘Eye of Providence’, blurring the business lines that kept them distinct from one another in the past.
There are currently five LLMs that are at roughly the same stage of development as the ChatGPT 4.0 class of model: OpenAI supported by Microsoft, Anthropic supported by Amazon, Google’s Gemini, Meta’s Llama and xAI’s Colossus. There are others but these seem to be the leaders from an efficiency point of view – which is measured using the metric Model FLOPS (Floating Point Operations) Utilisation or MFS for short. FLOPS is a fast and easy way to understand the number of arithmetic operations required to perform a given computation. MFS is the ratio of the observed throughput (tokens-per-second) relative to the theoretical maximum throughput of a system operating at peak FLOPs[1]. The more efficient the system the more you will be able to differentiate your model from the others and the greater your competitive advantage will become.
However, in order to break the deadlock and progress a model’s capability, scaling laws will have to hold true. So far scaling laws – how a model’s quality evolves with increases in its size, training data volume, and computational resources – have held up, but concerns are starting to creep in that we may be reaching the limits. GPT5 was meant to have been released by now, but model developers have not been able to deliver the game-changing developments that promise to usher in a new era of AI-powered productivity and creativity. Despite OpenAI releasing Strawberry, a new iteration of OpenAI’s LLM that will power GPT5, there is growing scepticism that we will see GPT5, with its promised order of magnitude improvements, before the end of next year, let alone this year.
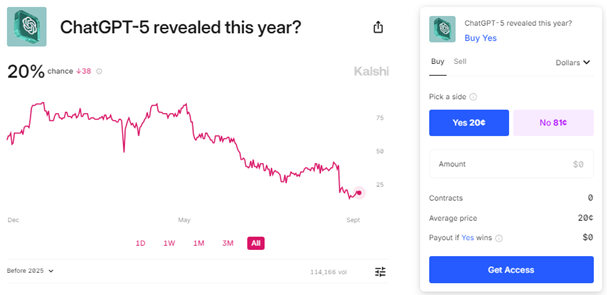
The fact that none of the competitors have managed to deliver their next iteration suggests real-world problems are getting in the way of scaling laws. So far, compute power has been enhanced by clustering GPUs together. Elon Musk claims that xAI’s Colossus AI training cluster, which comprises 100,000 NVIDIA H100 GPUs, is now live, making it the world’s largest GPU cluster. Getting bigger clusters working coherently and delivering greater compute power would require more powerful chips, improved networking, better memory, and superior data centre layouts facilitating novel AI cluster architectures and an ever-greater consumption of energy. Scaling, to say the least, has its headwinds!
But this isn’t deterring those in control. Scaling, in their minds, is achievable until there is irrefutable proof it isn’t, and investment will continue to ramp across the ecosystem irrespective of whether the near-term returns justify the gambit. The stakes are too great, in their minds, not to be in the race. Because they are not necessarily looking at immediate payoffs – coupled with the threat of a winner-takes-all endgame - the risks to Mag-7 investors increase as the game gets more expensive to play.
Even if scaling is possible there is a risk that the main players are unable to maintain the pace of development anticipated by investors, which may have huge ramifications on the current investment cycle within the AI ecosystem. Ever since the yen carry trade implosion in early August the semiconductor industry has, as a whole, lagged the recovery.

Should any of the current LLMs show scaling is possible and deliver the next breakthrough in model quality and utilisation, the current pull-back in AI infrastructure and semiconductors will look like a good topping-up opportunity. The longer we have to wait the deeper the current pull-back may become. For the less informed it all seems a bit binary.
Are investors already moving on from the idea of an all-seeing, all-knowing digital God? I’ll let you decide…
For those looking for further insight into this debate, I highly recommend you listen to this episode of Patrick O’Shaughnessy’s podcast Invest with the Best, where he interviews Gavin Baker.